Verbalising machine needs with data
Manufacturing companies strive for high productivity and minimal waste but some failures are out of their control. Professor George Panoutsos has developed a framework to understand the needs of machines and prevent these future failures.
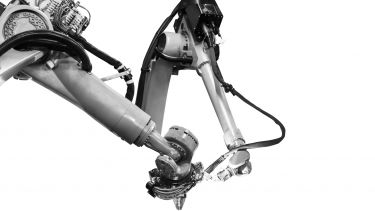
For new products to come to market the manufacturing process needs to be viable. This means that the item can be produced cheaply and efficiently enough to be profitable while minimising damage to the environment. A manufacturing process that creates defective products is wasteful, costly and can cause a downward spiral of reduced productivity before ultimately closing a business.
Quality control is a key component of keeping the costs of manufacturing to a minimum. It involves testing units to ensure that they fit within the required product specifications.
Manufacturing is becoming more advanced with improved sensors and specialised information available about the machines themselves that generate important data. This data is collected to provide information about the machinery and help firms track and monitor any quality inconsistencies. However, even for some of the most sophisticated data systems, it is very challenging to predict or prevent machine failures.
While modern quality control measures have become increasingly complex, manufacturing processes are also becoming more advanced, integrated and highly specialised.
Today, more data is collected about the production process and its machinery than ever before. The problem now is finding novel ways to monitor and understand this vast data and use it to deliver optimal manufacturing conditions.
For Professor George Panoutsos from the Department of Automatic Control and Systems Engineering and his team, this additional data is less of a problem and more of an opportunity.
The team at 91直播 have revolutionised precision manufacturing by developing the Self Learning System. This innovative system uses data to understand how machinery performs and expresses this in linguistic terms to predict future possible defects and failures. The autonomous linguistic interpretability of the system creates a human-centric framework that can be easily understood by the end-users.
91直播鈥檚 Self Learning System
Working closely with a team of post-doctoral and PhD researchers, George worked within European and UK Research and Innovation (UKRI) funded projects to develop a system that fundamentally changed the manufacturing sector of high precision laser-based joining by giving machines a voice.
鈥淚f a company starts generating product defects, even if it鈥檚 only two or three per cent, it means that you have to amend the manufacturing process. This is costly for the business and could prevent them from entering the market. The main way for a company to have a strong business case is to have security in a zero-defect manufacturing process and the Self Learning System (SLS) is a key part of enabling this鈥 explains George.
With a wealth of experience in computational intelligence, data-driven modelling and process optimisation, George collaborated with industrial partners to test and develop the SLS.
Through prototypes, the team captured data in a series of models to make robust predictions about where operative failures might occur and where machine operation could be optimised. The team worked closely with leading industrial manufacturers, such as Hidria (a world-leading corporation in automotive and industrial technologies) and LORTEK (a private technology centre) to combine the industry鈥檚 state of the art monitoring systems with George鈥檚 mathematical models and test its real-time interpreted analysis.
鈥淭he real-time functionality is vital for the learning stage and mostly exists to help the system quickly adapt and understand the manufacturing process behaviour. This data generates information into the form of linguistic statements and allows it to be read easily by anyone on the production line, rather than waiting for a specific expert to come in. From there it uses data driven mathematical models, or a set of equations, to forecast future machinery behaviour鈥 says George.
鈥淭raditionally, the data would have been visualised as numbers or plots on a graph. The SLS summarises this data into statements such as 鈥榠f X is too high then Y will stop functioning鈥. This provides clear linguistic commands and makes the information accessible to a range of audiences, rather than solely for experts鈥 adds George.
The SLS exploits state-of-the-art research in Computational Intelligence to develop a machine learning model that uses manufacturing process data to learn how a process performs and predicts future defects and failures. The advantage of this system lies in its structure and presentation of data. Its data-driven approach requires minimum-to-no user intervention and can be interpreted by non-experts.
Today, the technology is being implemented at an industrial scale. Through applications in the automotive, oil and gas, and white goods sectors the SLS is revolutionising precision laser welding.
An industry breakthrough
In 2017, , a leading international provider of customised solutions for automotive and industrial applications, adopted the SLS in their welding manufacturing processes.
Our aim was to optimise the speed of the manufacturing process while minimising defects produced and this is where we鈥檝e had the biggest impact. Hidria originally had low for the sector yield rate in pilot production lines. After using the SLS, supported by technology and process development within a European research programme the yield turned to commercially viable and marketable levels
Jani Kenda
Development and Project Technology Manager at Hidria
The SLS, combined with other technology advancements emanated from the European programme, dramatically improved the production process in terms of quality and durability of the welds and enabled an 80 per cent reduction in scrap, 30 per cent productivity increase and five per cent improvement in process speed.
The successful influence of the SLS allowed the commercialisation of an award winning pressure sensor glow plug, a device designed to improve engine efficiency. Manufactured effectively using the SLS, the sensor is now found in a third of modern diesel engines in Europe and lowers emissions by 30 per cent.
The research was instrumental to the delivery of an environmentally sustainable solution to the diesel engine market and the team hope to adapt the model to other manufacturing processes.
Future predictions
Manufacturing is a highly competitive industry and it will likely continue to be, particularly with the rise of countries who can produce products at a much lower cost. It鈥檚 therefore vital that companies maintain high yield rates and as close to zero-failures as possible.
Over time the models and framework will need updating as technology continues to advance.
鈥淚t鈥檚 the greatest weakness and the greatest strength of data-driven models. They rely on data so if the process changes the resulting framework will need to be different,鈥 says George. However, this can be supported by research in adaptive and evolving data-driven systems.
鈥淲e are now looking at applying the SLS to the growing industry of 3D printing, and in particular metal additive manufacturing. The university is a national centre (EPSRC Manufacturing Hub) for powder-based manufacturing, this is a sector we want to work with. The underpinning process monitoring, optimisation and control requirements are not too dissimilar and will help us understand how the SLS can work with non-traditional manufacturing systems鈥 explains George.
鈥淧art of my current research is focussing on how to extend this work to accommodate more high resolution data; this will represent a further challenge on how to structure and interpret the system model, across different sensing systems and modalities. We鈥檙e working to provide more ways for the system to interpret and understand data and create additional linguistic phrasing as the nature of data becomes more complex鈥 he adds.
George鈥檚 manufacturing solution is the first step to verbalising the internal state of manufacturing processes in high precision welding. Such systems, when used in advanced manufacturing more broadly, have the potential to adapt to technologies, reduce waste and develop opportunities for innovative products as they come to market.
Written by Alina Moore, Research Communications Coordinator