PhD Study
Funded PhD projects available at the Healthy Lifespan Institute
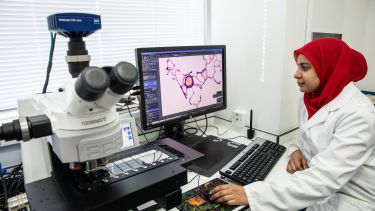
These 3.5 year studentships are part of the Healthy Lifespan Institute at 91Ö±²¥. The Institute is dedicated to the understanding and prevention of multimorbidity, which is the simultaneous presence of multiple chronic health conditions. Using a multidisciplinary approach, we aim to help people live longer, healthier and more independent lives.
As a student, you will be a valued and active member. You will be part of a wider network of PhD students and will have the chance to influence the Institute, to take part in seminars and events, and to meet leaders in the field.
Identifying determinants of co-morbidities in patients affected by osteoarthritis using machine learning
As people live longer, the number of people with multiple diseases and complex medical needs has dramatically increased. As diseases are treated individually, problems of polypharmacy and high costs appear. In the UK, age-related multimorbidity already costs 45 billions/year and it is on the rise.
The study of multimorbidity is complex, and poses excellent methodological challenges in the analysis of temporal event data sequences. On the data access and availability side, healthcare providers routinely record longitudinal data for all patient encounters. These datasets are large and underexploited. On the methodological side, machine learning dominates the formulation of data-driven predictive models and has shown its benefits in a diverse range of applications.
To start unfolding this complexity, this PhD studentship will focus on osteoarthritis (OA), a disease associated with ageing, and a high number of comorbidities.
The hypothesis is that it is possible to identify subgroups of OA patients which will develop one or more comorbidities with a similar longitudinal sequence and that for these subgroups it is possible to identify determinants or predictors of those comorbidities.
Molecular mechanisms driving chronic macrophage dysfunction in ageing
Macrophages are involved in self-defence against pathogens and in tissue homeostasis and repair. They are important to detoxify tissues by clearing apoptotic cells and modified lipids. In response to environmental cues, macrophages are able to shift from an inflammatory to a reparative phenotype. Whilst it is known that macrophage function declines with old age, little systematic work has been published that directly investigates the molecular mechanisms of age-related changes in human primary macrophages.
This project combines complementary interests in macrophage biology and their role in immuno-metabolic diseases, machine learning, and modelling transcriptional dynamics of activated macrophages. We will study monocyte derived macrophage (MDM) responses from healthy young adults vs aged human subjects.
A systems biology approach, combining mathematical modelling and computational biology with experimental data will be used to characterise the dynamic transcriptional landscape of macrophage activation. Pathway analysis tools, discrete-logic modelling and optimization will be used to infer from data contextualised Prior Knowledge Network (PKN) models of macrophage activation and lipid processing to predict missing expression values, analyse network stability or to simulate transitions between macrophage activation states in response to perturbations.
How do health-related behaviours cluster within individuals and how does this change over time, across the life course and between birth cohorts?
Given that everyday life involves many behaviours that affect multimorbidity, it is crucial to understand how behaviours relate to one another, both to develop our understanding of behaviour and to inform intervention strategies. This studentship aims to understand how health-related behaviours associated with future multimorbidity cluster within individuals (e.g. to what extent is drinking alcohol associated with smoking and / or reduced physical activity?), with a focus on whether and how clustering changes over different temporal process (e.g. across the life course, between birth cohorts, or across the whole population over time).
Acquisition and analysis of real-world sensing data to aid prediction, diagnosis and monitoring of cardiovascular disease
A major obstacle to prevention, diagnosis and treatment of chronic cardiovascular diseases is the lack of medically relevant data obtained in daily life . The increasing availability of cheap, scalable technologies to collect long-term, real-world behavioural, physiological and environmental data from patients during daily life, when combined with computer modelling, artificial intelligence, and machine learning provides an opportunity to radically improve patient care. This requires collaboration between physicians and experts in acquisition and analysis of complex datasets.